NVIDIA PhysicsNeMo
NVIDIA PhysicsNeMo is an open-source python framework for building, training, and fine-tuning physics AI models at scale.
NVIDIA PhysicsNeMo provides utilities that enable developers to build AI surrogate models that combine physics-driven causality with simulation and observed data, enabling real-time predictions. From Neural operators, GNNs to generative AI models, developers can develop proprietary AI models to enhance engineering simulations and generate higher-fidelity data for scalable, responsive designs. NVIDIA PhysicsNeMo supports the creation and validation of large-scale digital twin models across various physics domains, from computational fluid dynamics and structural mechanics to electromagnetics.
Use NVIDIA PhysicsNeMo to bolster your engineering simulations with AI. You can build and validate models for enterprise-scale digital twin applications across multiple physics domains, from computational fluid dynamics and structural to electromagnetics.
Download NowDocumentationNVIDIA PhysicsNeMo GitHubData Sheet
Physics-Informed Machine Learning for Surrogate Models
What’s New In NVIDIA PhysicsNeMo
NVIDIA Blackwell Accelerates Computer-Aided Engineering Software Ecosystem
Leading Software Providers Including Ansys, Altair, Cadence, Siemens and Synopsys Adopt NVIDIA Blackwell
Leading Climate Tech Companies Adopting Earth-2 for Faster, More Accurate Predictions and Disaster Preparedness
Abu Dhabi-Based AI Company G42, JBA Reinsurance, AXA Insurance Among First to Adopt NVIDIA Omniverse Blueprint for Earth-2 Weather Analytics.
Features
PhysicsAI Model Architectures
NVIDIA PhysicsNeMo offers a variety of approaches tuned for training physics-AI models, from purely physics-driven models like physics-informed neural networks (PINNs) to physics-based, data-driven architectures, such as neural operators, graph neural networks (GNNs), and generative AI-based diffusion models.
NVIDIA PhysicsNeMo includes curated physics-ML model architectures, Fourier feature networks, Fourier neural operators, GNNs, point cloud and diffusion models trained on NVIDIA DGX? across open-source, free datasets found in the documentation.
Training State-of-the-Art Physics-AI Models
NVIDIA PhysicsNeMo provides an end-to-end pipeline and utilities for training physics-ML models—from ingesting geometry to adding partial differential equations. NVIDIA PhysicsNeMo also includes training recipes in the form of reference applications. View documentation.
Training at Scale
NVIDIA PhysicsNeMo provides GPU accelerated distributed framework to build foundational scale models. It provides data parallel and model parallel training pipelines scaled to multi-node training to suit the needs of industrial scale problems. For instance, developers can train a GNN or a point cloud on a 50 million node mesh quite easily.,
PhysicsAI Reference Pipelines
NVIDIA PhysicsNeMo offers a diverse set of reference pipelines as a starting point for developers to customize and build their own solutions. These reference samples show how one can model a specific problem with AI, showcases some relevant architectures and datasets. This spans use cases from CFD, thermal analysis to climate and weather. View the github repo, github for the complete list.
Benefits
NVIDIA PhysicsNeMo is an open-source, freely available AI framework for developing physics-ML models and novel AI architectures for engineering systems.
AI Toolkit for Physics
Quickly configure, build, and train AI models for physical systems in any domain, from engineering simulations to life sciences, with simple Python APIs.
Customize Models
Download, build on, and customize state-of-the-art pretrained models from the NVIDIA NGC? catalog.
Near-Real-Time Inference
Deploy AI surrogate models as digital twins of your physical systems to simulate in near real time.
Scale With NVIDIA AI
Leverage NVIDIA AI to scale training performance from a single GPU to multi-node implementations.
Open-Source Design
Experience the benefits of open source. PhysicsNeMo is built on top of PyTorch and is released under the Apache 2.0 license.
Standardized
Work with the best practices of AI development for physics-ML models, with an immediate focus on engineering applications.
User Friendly
Boost productivity with user-comprehensible error messages and easy-to-program Pythonic API interfaces.
High Quality
Use high-quality software with enterprise-grade development, tutorials for getting started, and robust validation and documentation.
Contribute to NVIDIA PhysicsNeMo Development
NVIDIA PhysicsNeMo provides a unique platform for collaboration within the scientific community. Domain experts are invited to contribute and accelerate physics-ML across a variety of use cases and applications.
See NVIDIA PhysicsNeMo in Action
Speed and Accuracy of Gen AI Helps Combat Climate Change
Accelerating Extreme Weather Prediction with FourCastNet
Siemens Energy HRSG Digital Twin Simulation Using NVIDIA PhysicsNeMo and Omniverse
Maximizing Wind Energy Production Using Wake Optimization
Accelerating Carbon Capture and Storage With Fourier Neural Operator and NVIDIA PhysicsNeMo
Predicting Extreme Weather Events Three Weeks in Advance with FourCastNet
Explore NVIDIA PhysicsNeMo Examples
Ways to Get Started With NVIDIA PhysicsNeMo
Containers and Models for Development
Develop physics-ML models using NVIDIA PhysicsNeMo container and pretrained models, available for free on NVIDIA NGC.
Computer Aided Engineering (CAE)
Explore how NVIDIA is enabling computer-aided engineering industry developers to accelerate physics-based CAE simulations and embrace real-time interactive design using AI-accelerated digital twins.
External Aerodynamics NIM
Very accurate pretrained model based on DoMINO architecture trained on a large and diverse automotive dataset and packaged as a NIM for performant and scalable cloud deployment. Developers can customize the model further using the training recipe in PhysicsNeMo.
Self-Paced Online Course
NVIDIA PhysicsNeMo provides explicit parameter specifications for training the surrogate model with a range of values to learn for the design space and for inferring multiple scenarios simultaneously.
Enterprise-Scale Workflows
NVIDIA PhysicsNeMo is available with NVIDIA AI Enterprise, an end-to-end AI software platform optimized to accelerate enterprises time to production, certifications to deploy AI everywhere, and enterprise-grade support, security, and API stability while mitigating the potential risks of open-source software
Omniverse Integration
Developers can use the Digital Twin for Interactive Fluid Simulation as a reference to couple surrogate models built in NVIDIA Phy
Higher Education and Research Developer Resources
Self-Paced Online Course
Take a hands-on introductory course from the NVIDIA DLI to explore physics-informed machine learning with NVIDIA PhysicsNeMo.
Access CourseTeaching Kit for Educators
A DLI Teaching Kit is available to qualified university educators interested in Physics-ML. Comprehensive and modular, the kit can help you integrate lecture materials, hands-on exercises, GPU cloud resources, and more into your curriculum.
Access Teaching KitWatch WebinarOpen Hackathons and Bootcamps
Accelerate and optimize research applications with mentors by your side.
End-to-End AI for ScienceHackathon GitHubUpcoming Open Hackathons
Explore More Resources
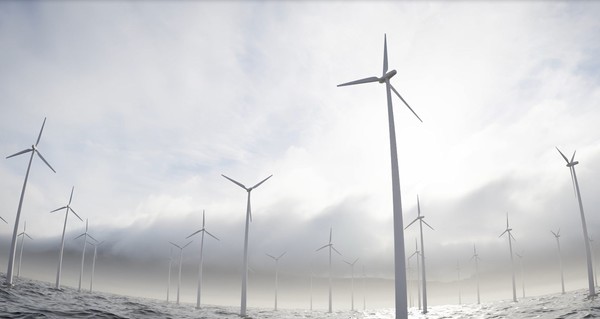
Using NVIDIA PhysicsNeMo and Omniverse Wind Farm Digital Twin for Siemens Gamesa
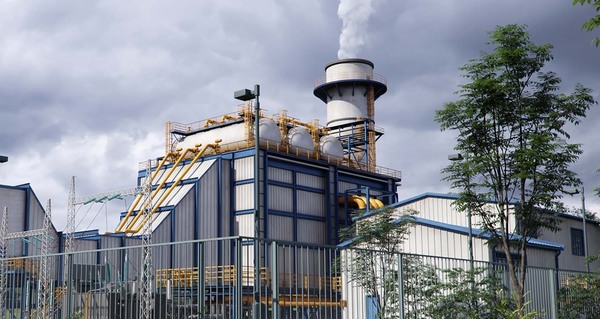
Siemens Energy Taps NVIDIA to Develop Industrial Digital twin of Power Plant in Omniverse and PhysicsNeMo
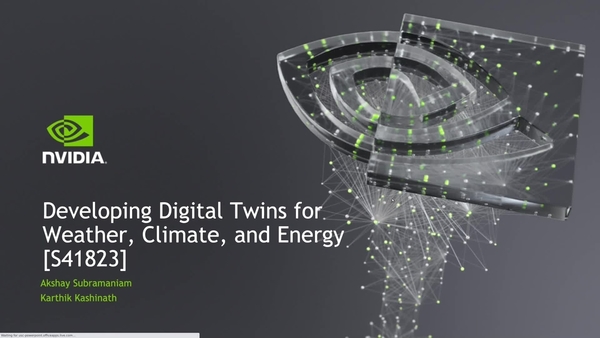
Developing Digital Twins for Weather, Climate, and Energy
Ethical AI
NVIDIA’s platforms and application frameworks enable developers to build a wide array of AI applications. Consider potential algorithmic bias when choosing or creating the models being deployed. Work with the model’s developer to ensure that it meets the requirements for the relevant industry and use case; that the necessary instruction and documentation are provided to understand error rates, confidence intervals, and results; and that the model is being used under the conditions and in the manner intended.